top of page
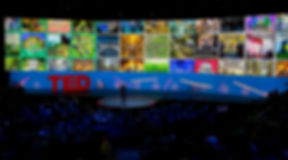
Noah Raford futurist Metaverse video games technology keynote Dubai Museum of the Future Future Foundation crypto blockchain AI politics security
bottom of page
Noah Raford futurist Metaverse video games technology keynote Dubai Museum of the Future Future Foundation crypto blockchain AI politics security